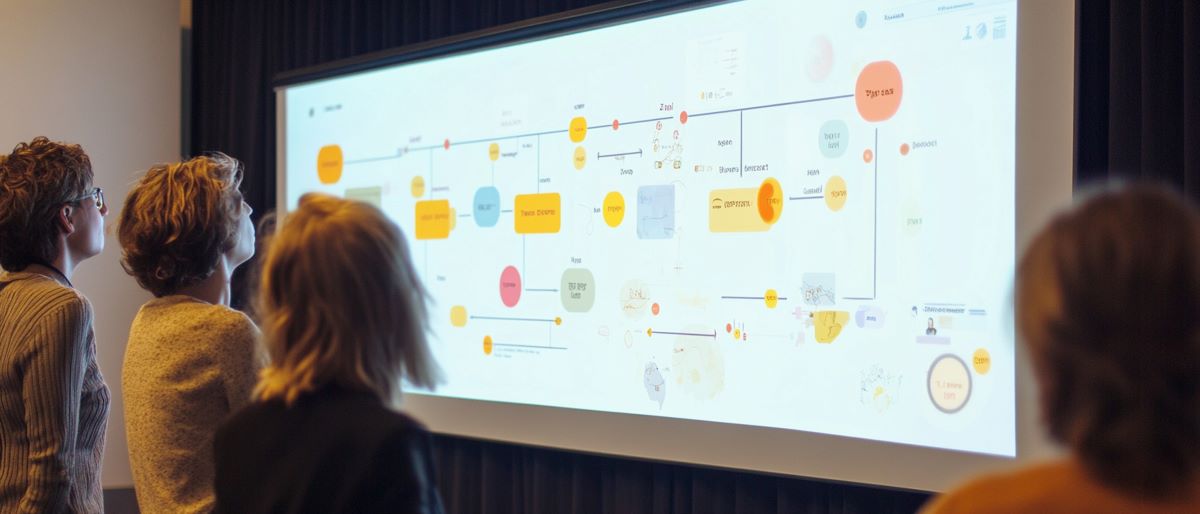
May 15, 2025
The Silent Advantage: How Proactive Journeys Beat Reactive Support
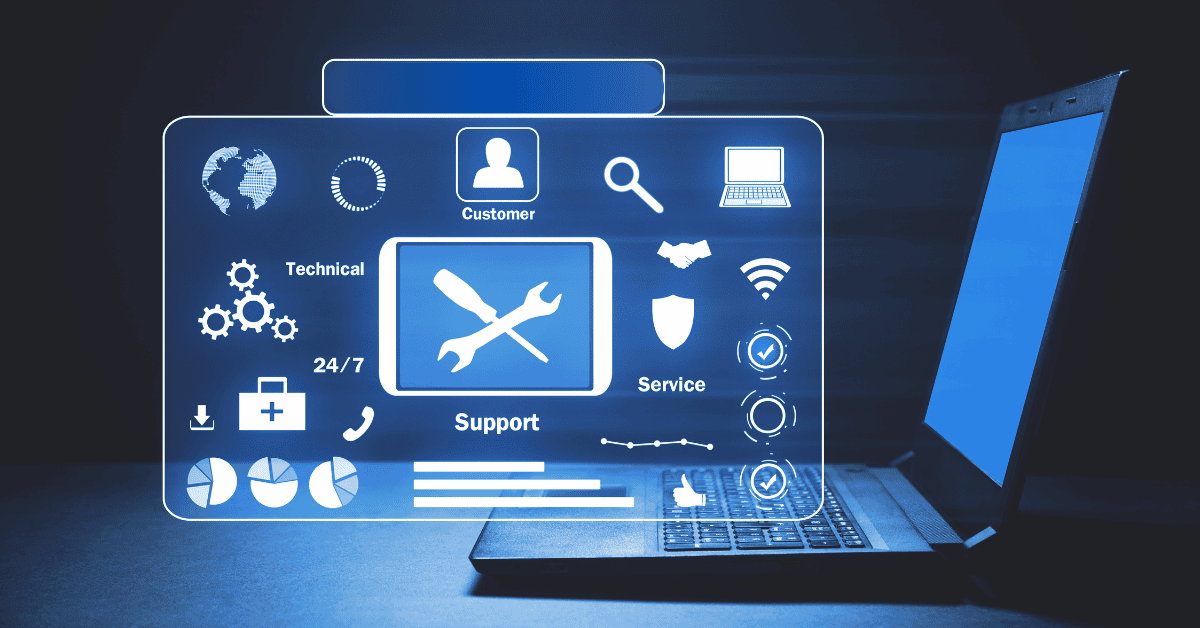
December 2, 2024
The Hidden Cost of Ineffective Contact Center Technology
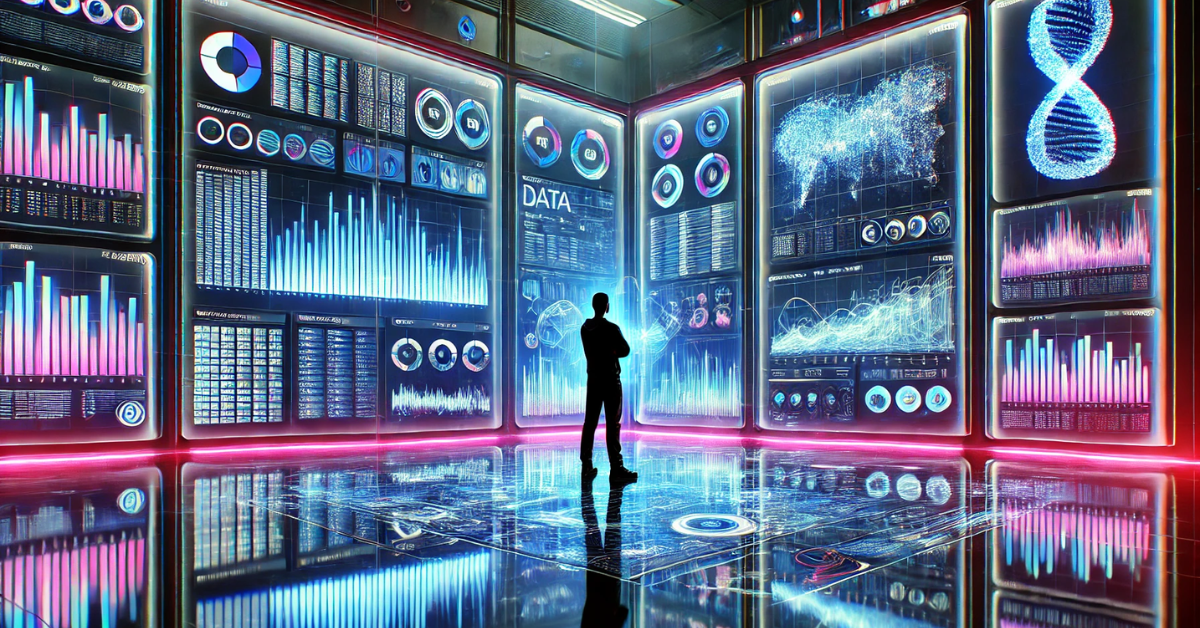
October 8, 2024
Data Governance in the Age of AI: A Competitive Edge for Business Leaders
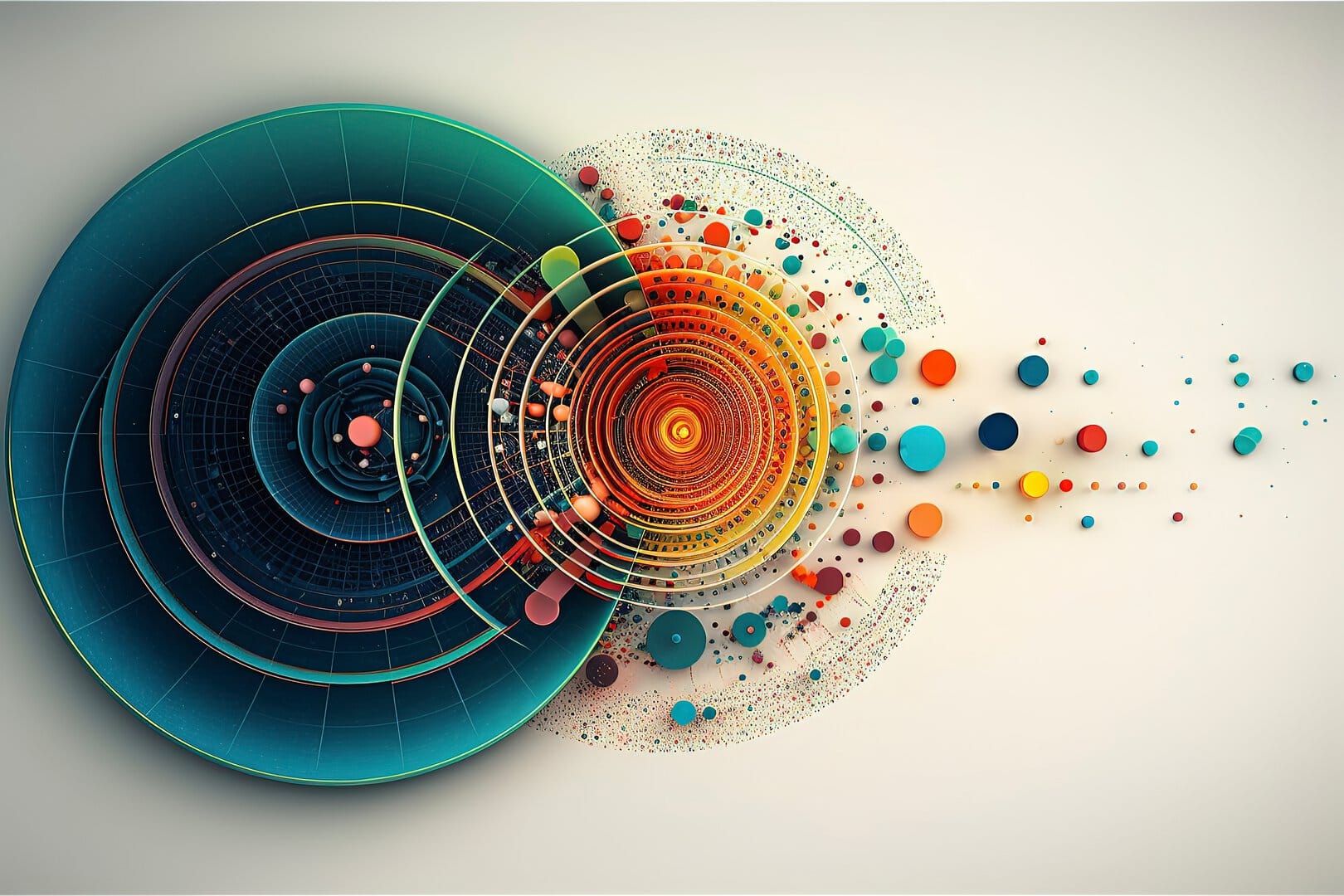
September 23, 2024
The Reality Gap: Sentiment Analysis vs. Customer Feedback
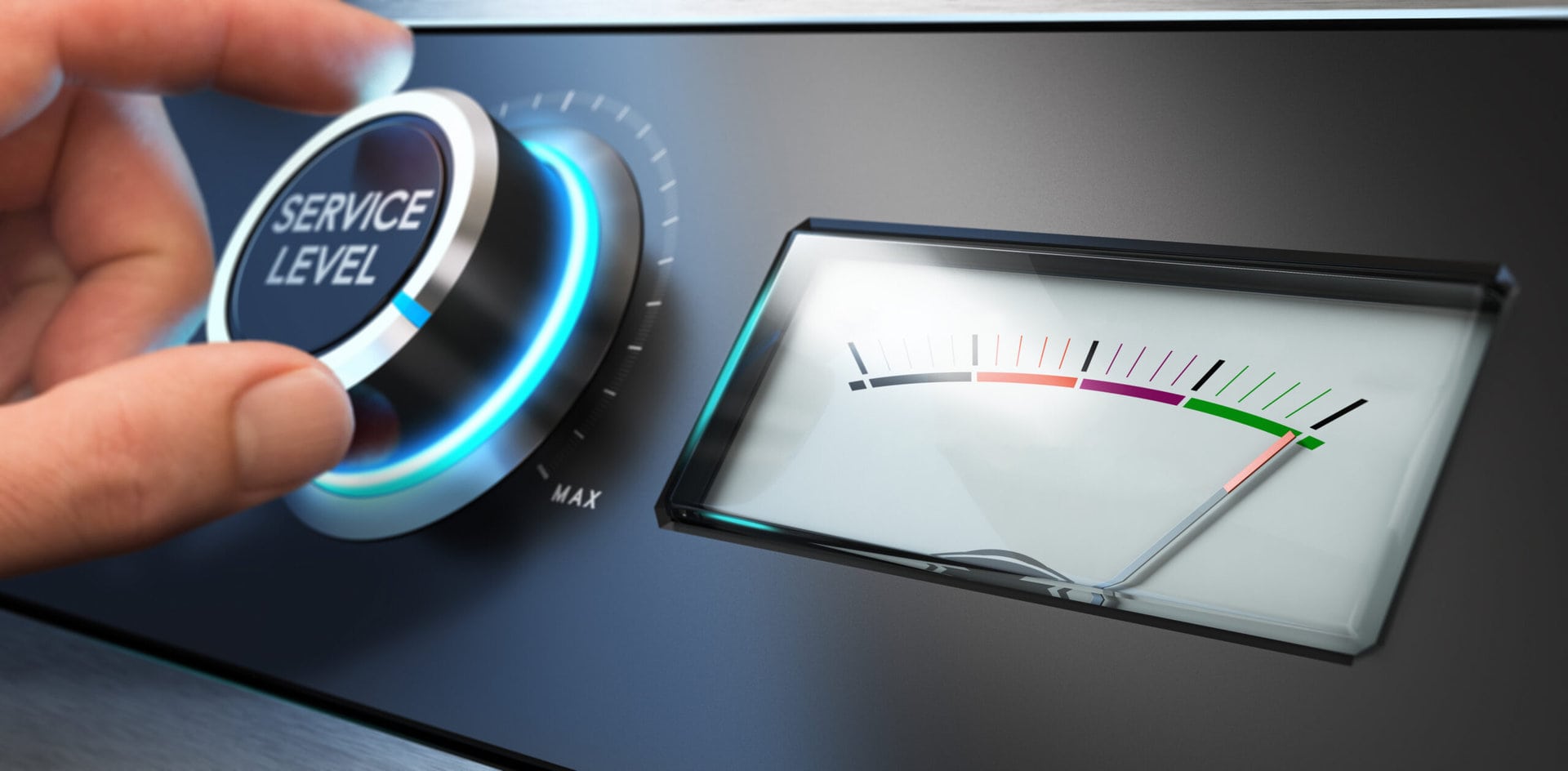
September 12, 2024
Service Level – Are You Measuring it the Wrong Way?
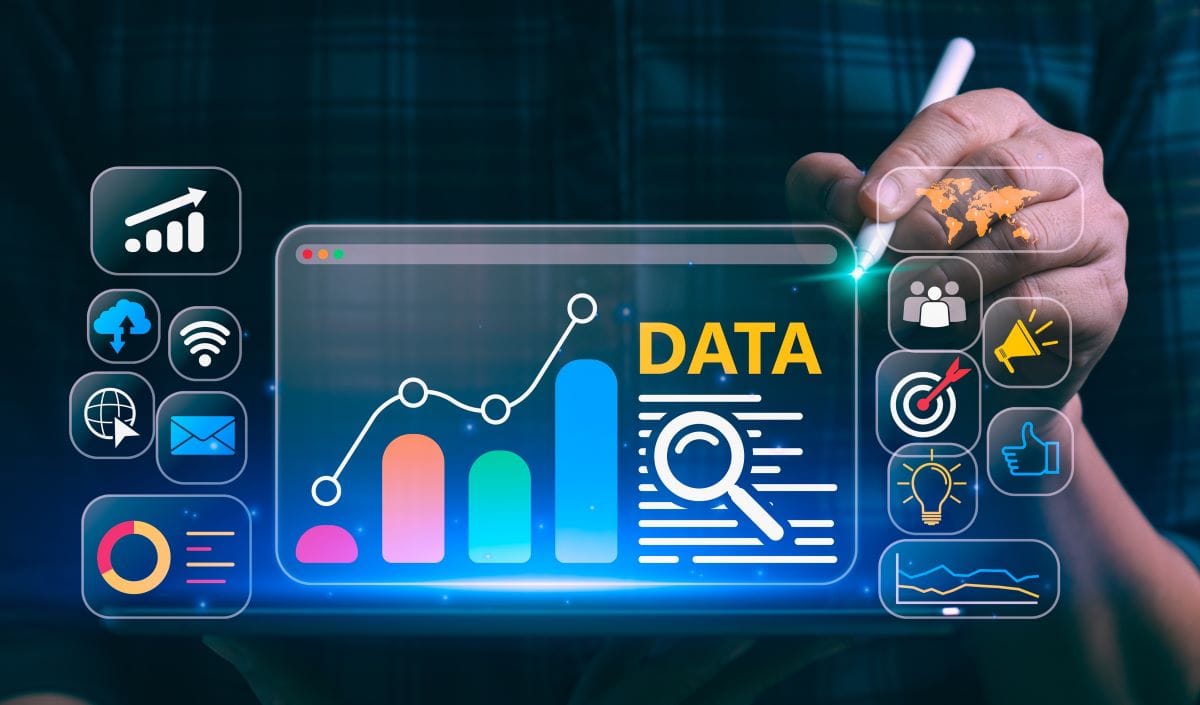
June 24, 2024
Analyzing the Current State of Customer Experience Amid Conflicting Data
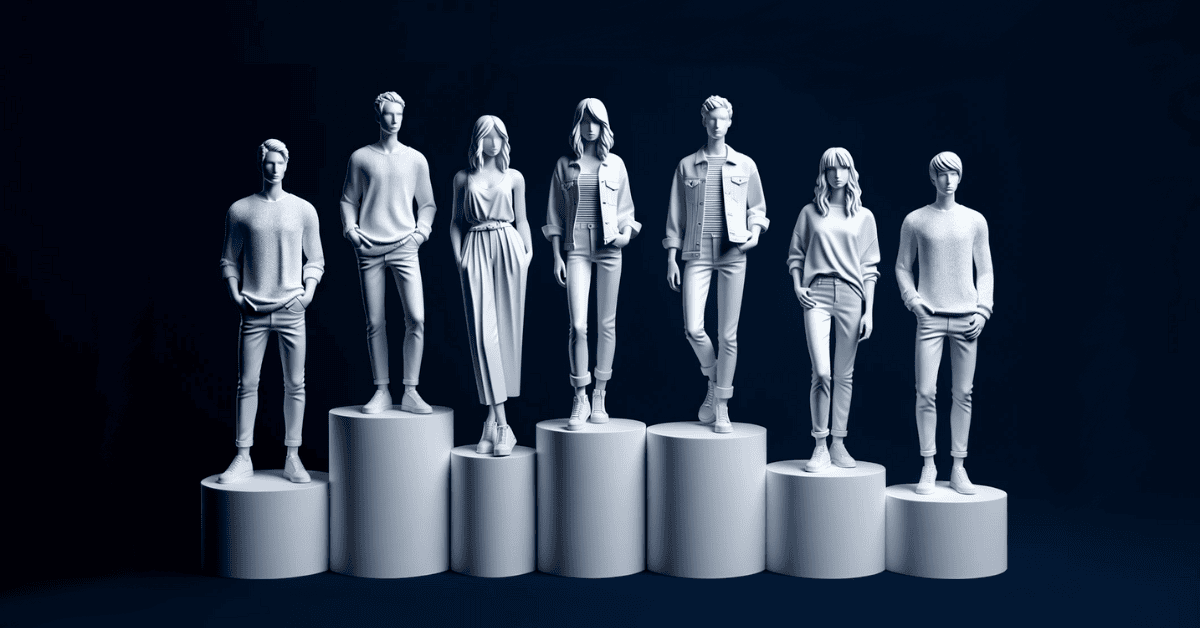
June 4, 2024
The Case for Recognizing Middle Performers
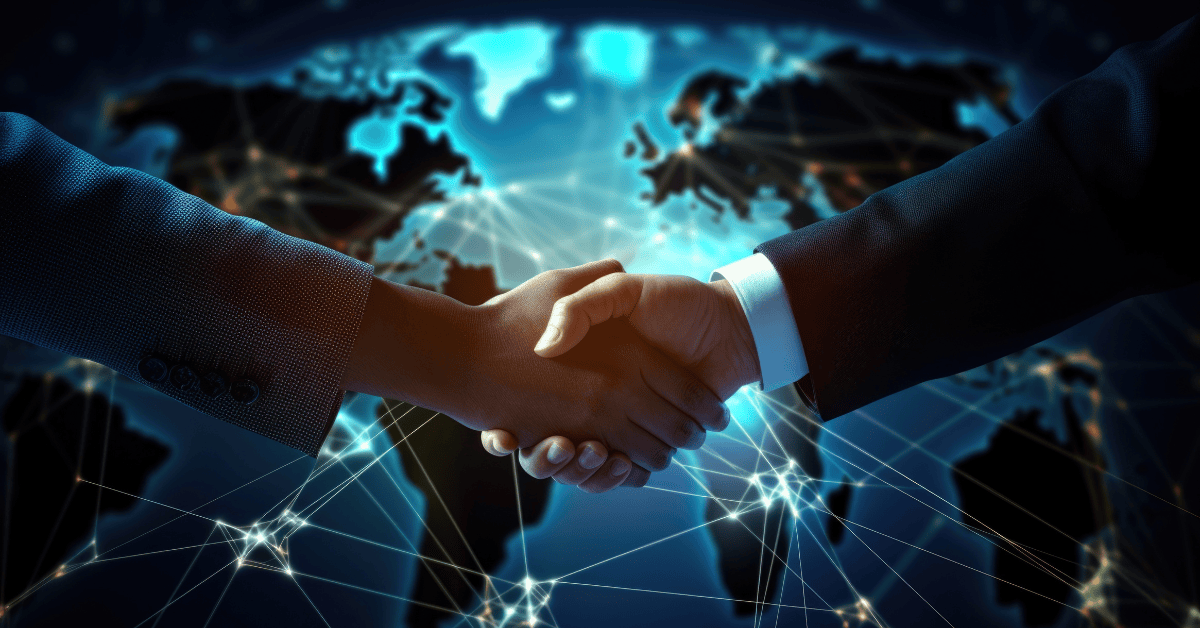
May 7, 2024
Contact Center Outsourcing: Selecting the Right Supplier
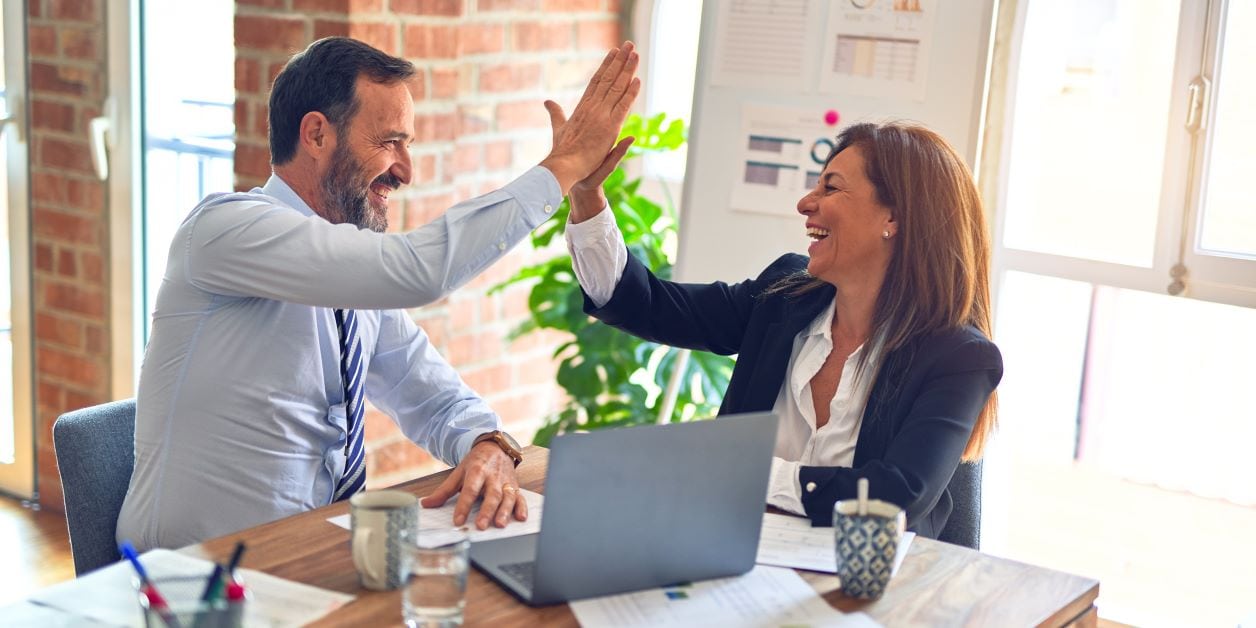
May 6, 2024
Quality Assurance and Customer Satisfaction: Three Ways to Ensure Alignment
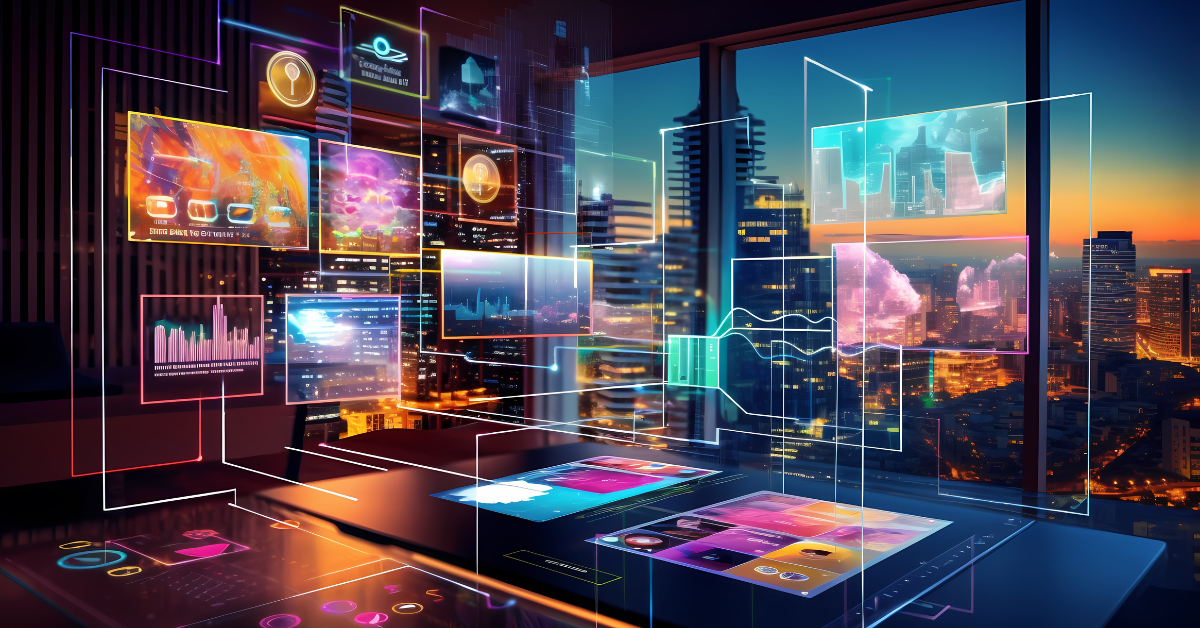
April 9, 2024
Seizing the Digital Future in Customer Experience Transformation
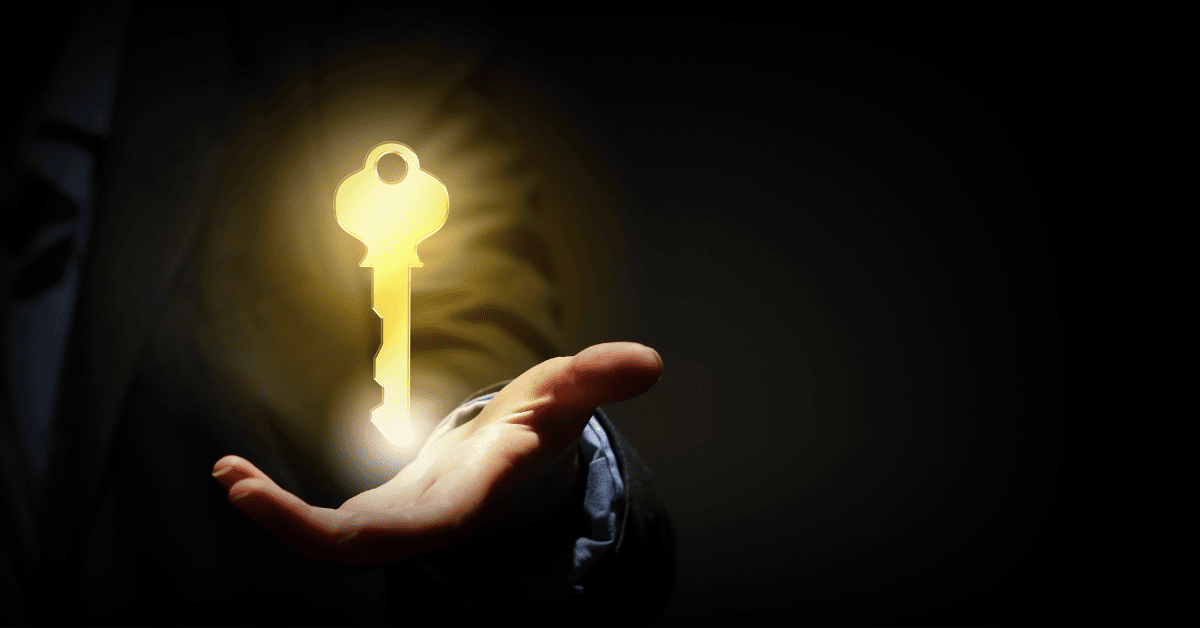
April 4, 2024
Contact Center Team Leaders: The Key To Engaged and Motivated Employees
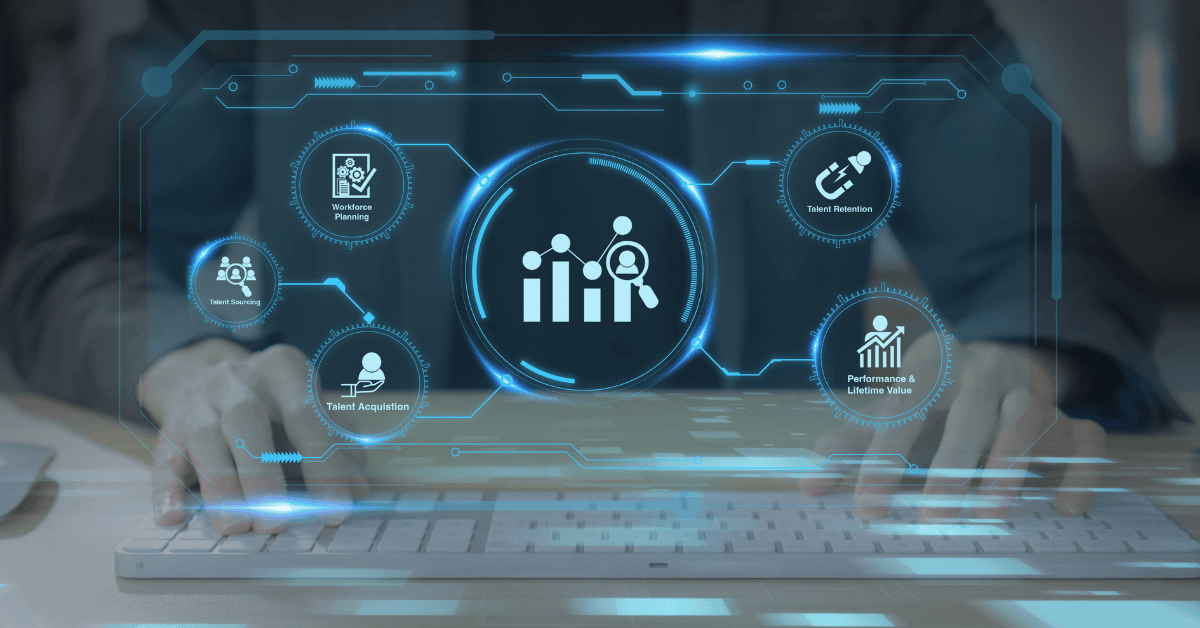
April 4, 2024
Training and Development as an Employee Retention Strategy
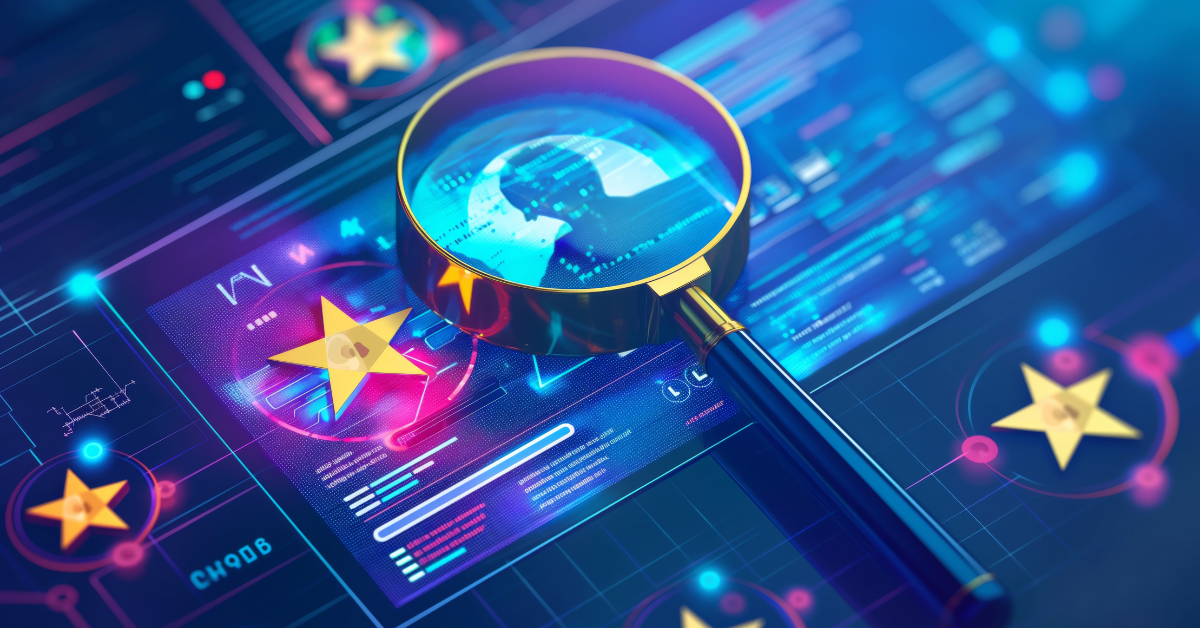
April 4, 2024
Employee Engagement and Retention: Consider This
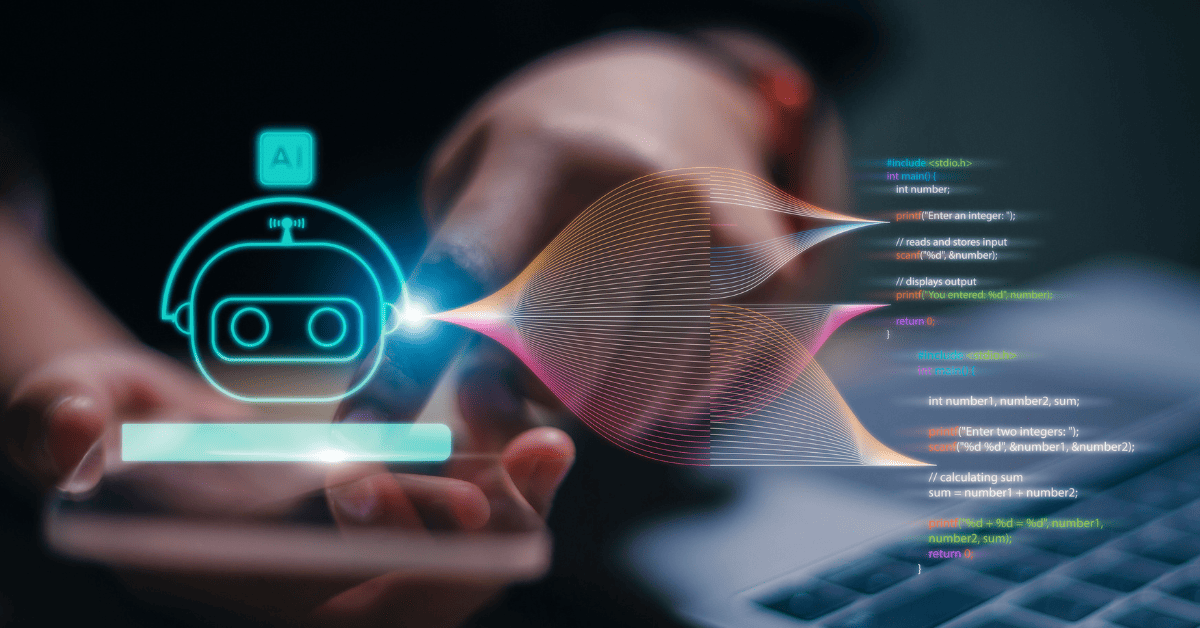
April 2, 2024
Customer Experience Technology: A Deep Dive into GenAI, LLMs and AI Assistants
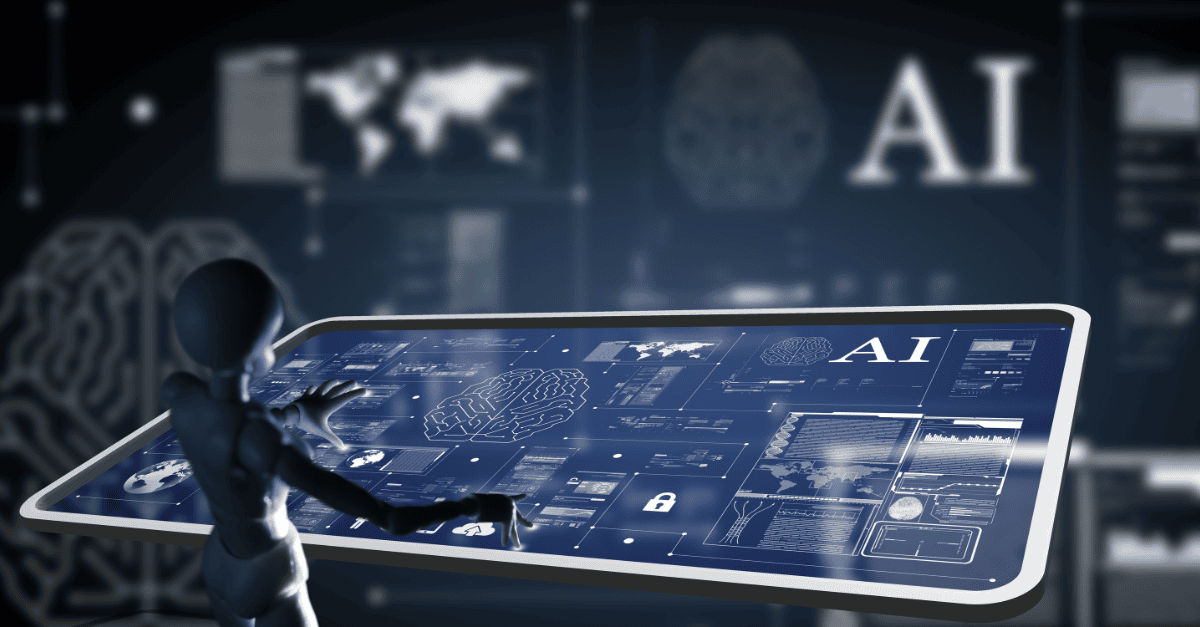
April 2, 2024
Implementing AI in CX: Strategies for Success
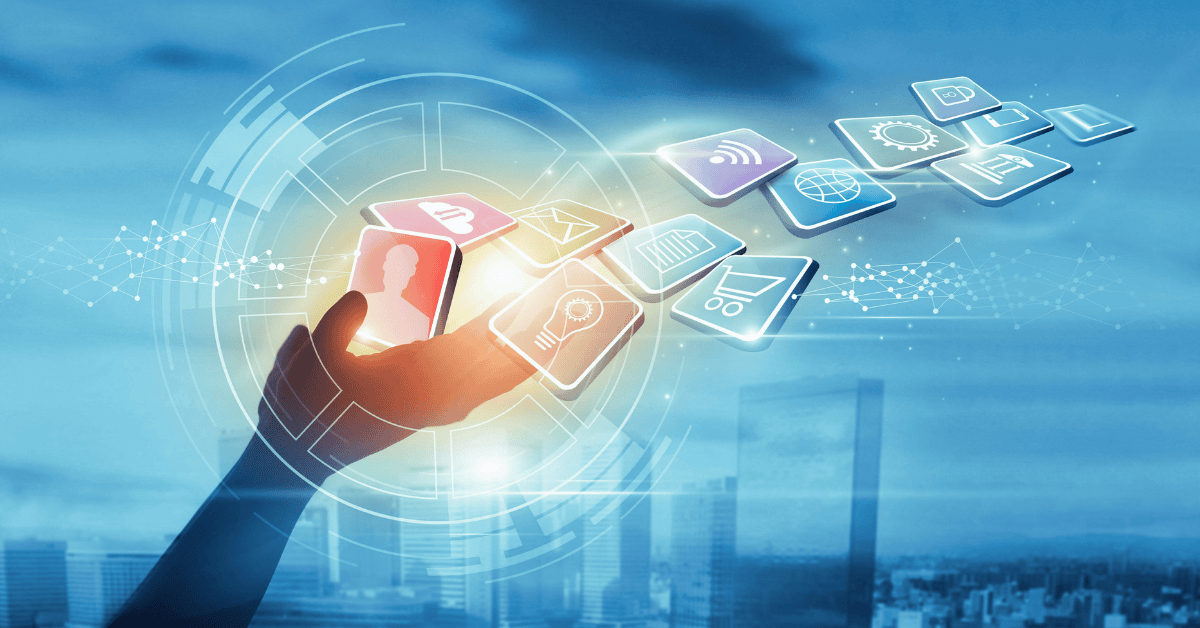
April 1, 2024
Self-Service Technology: A Customer-Centric Approach
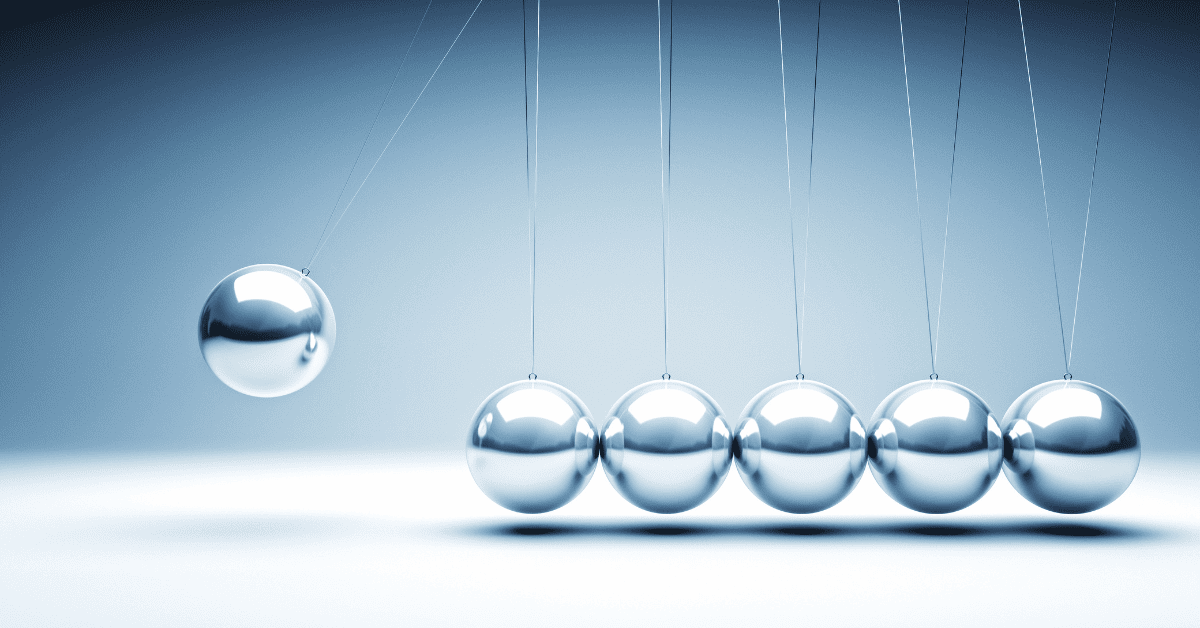
March 28, 2024
Using a Balanced Scorecard for Performance Management
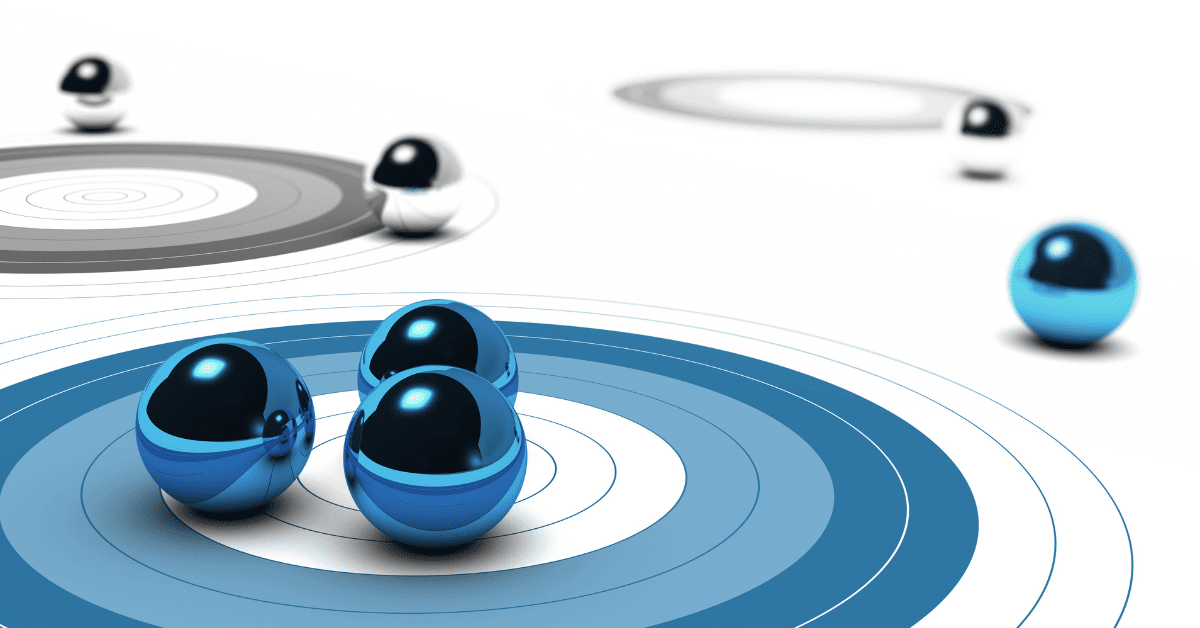
March 28, 2024
Creating a Balanced Scorecard: What to Consider
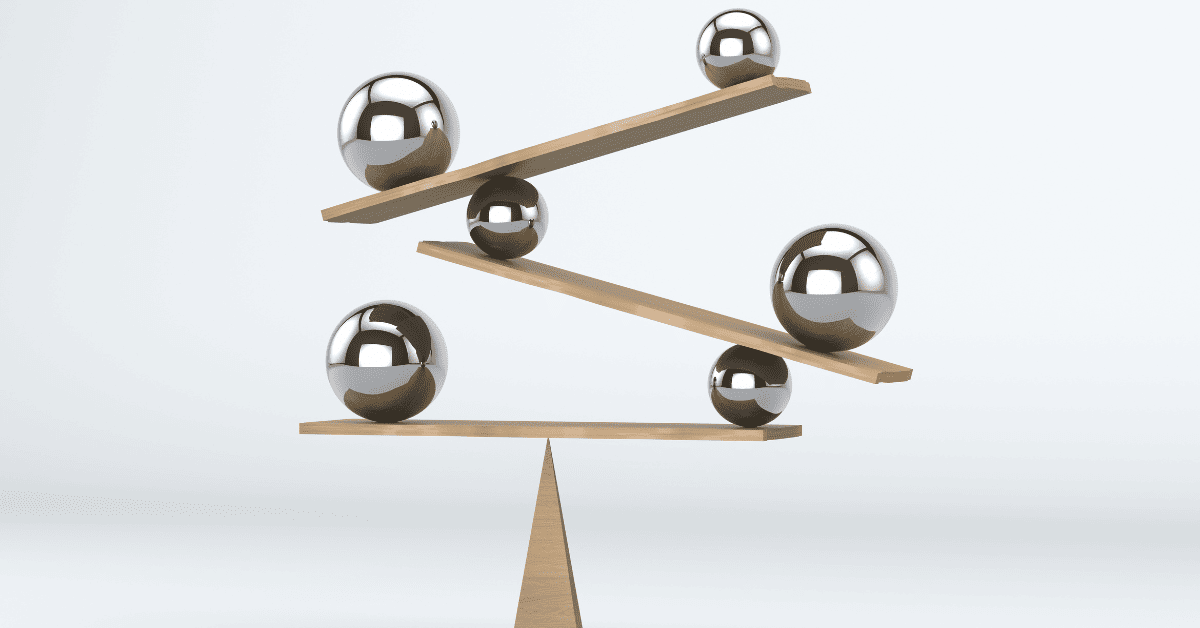
March 28, 2024
Benefits of a Balanced Scorecard for Performance Management
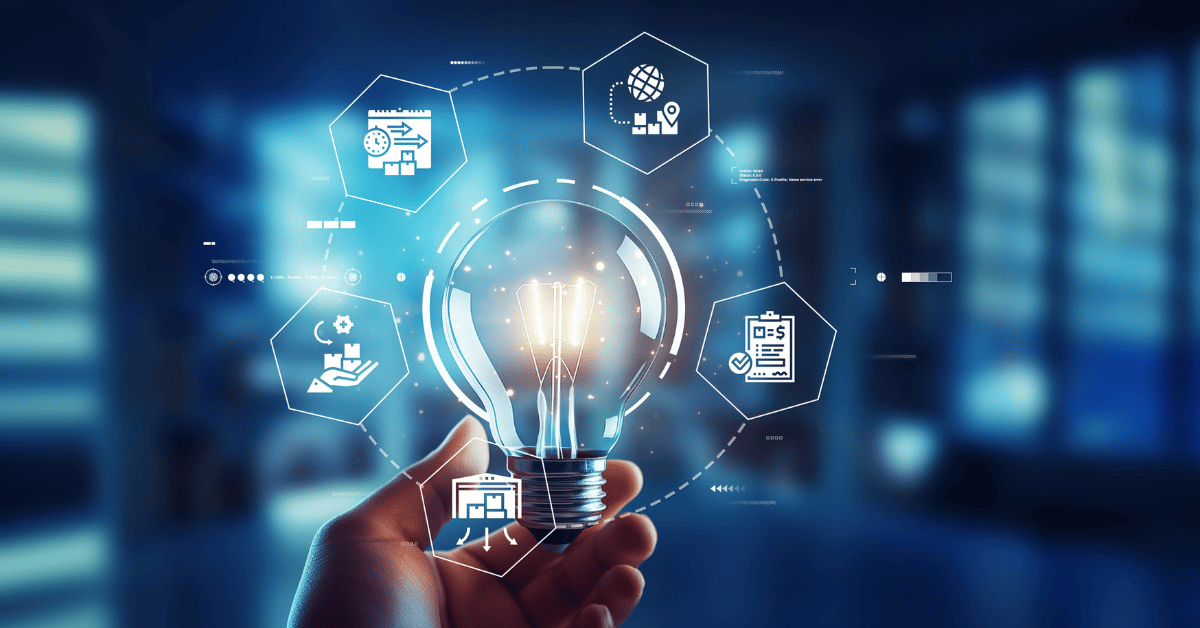
March 22, 2024
Developing a Strategic Vendor Management Framework
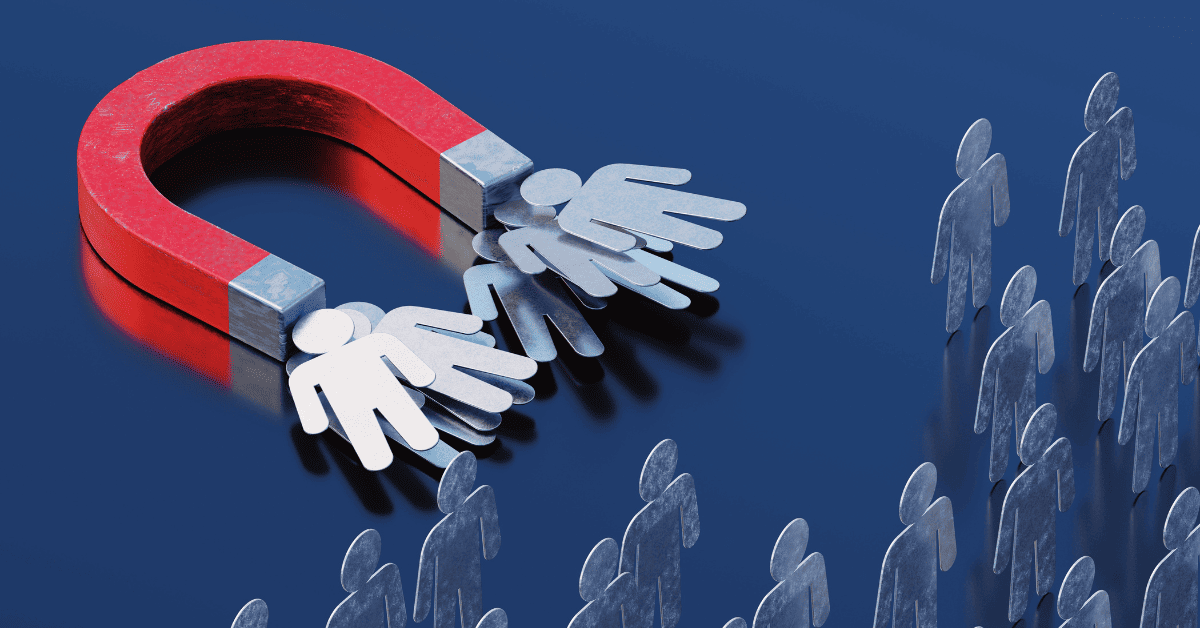
March 11, 2024
Leader’s Guide to Call Center Retention
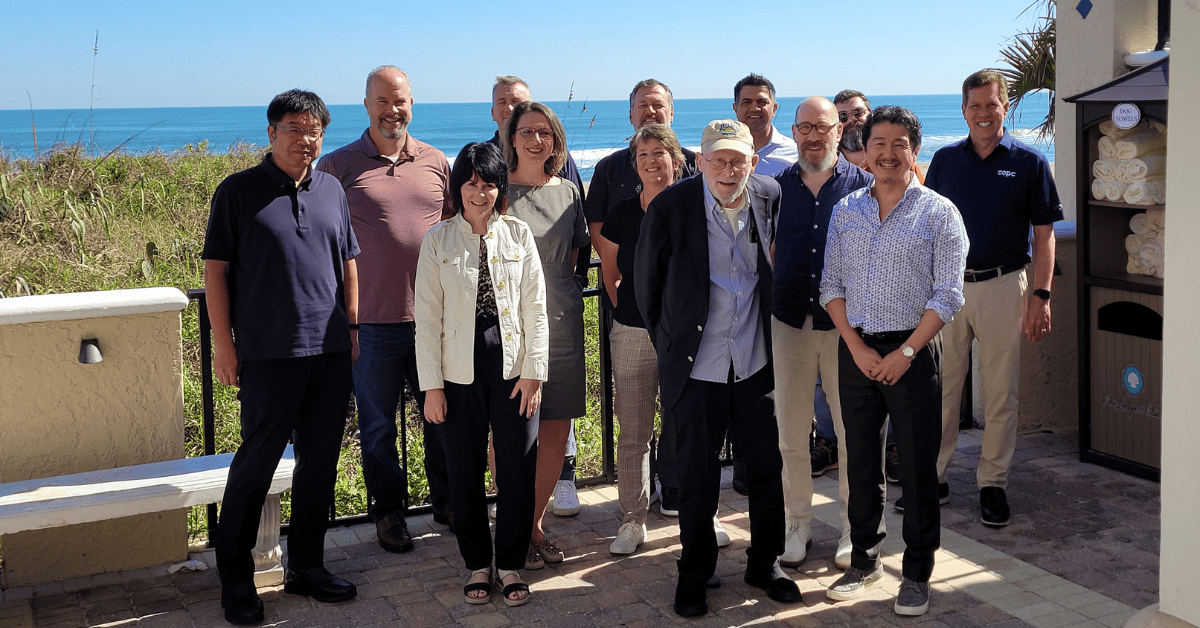
February 15, 2024
COPC Standards Committee Update: AI in Focus
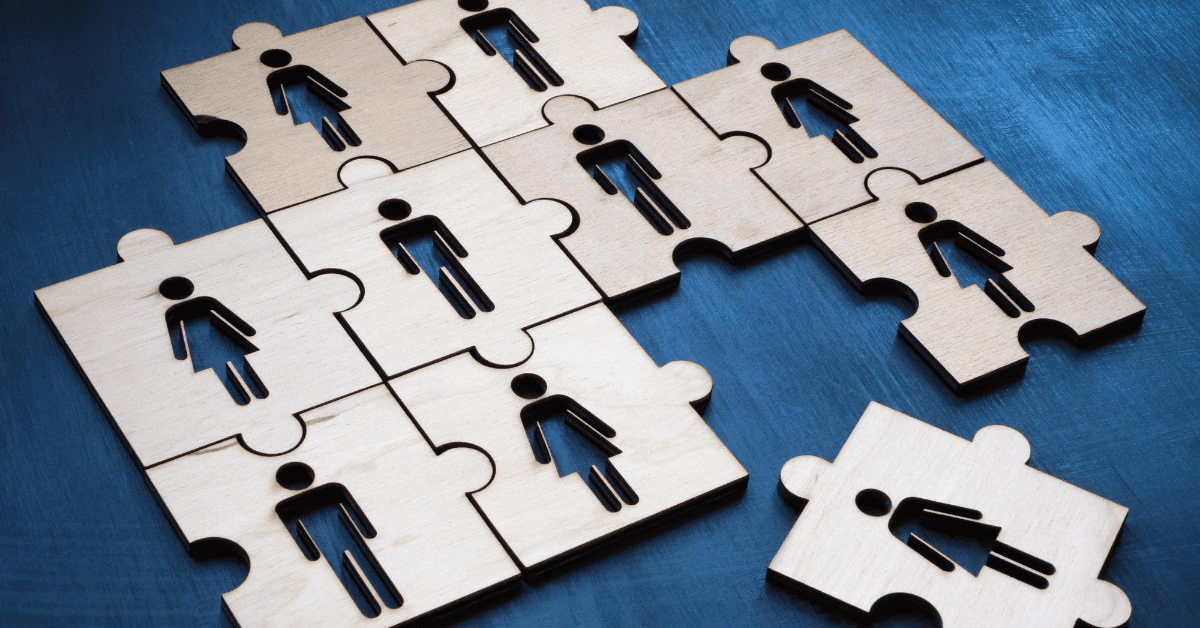
January 15, 2024
Onboarding Best Practices: Reducing Attrition in the First 90 Days
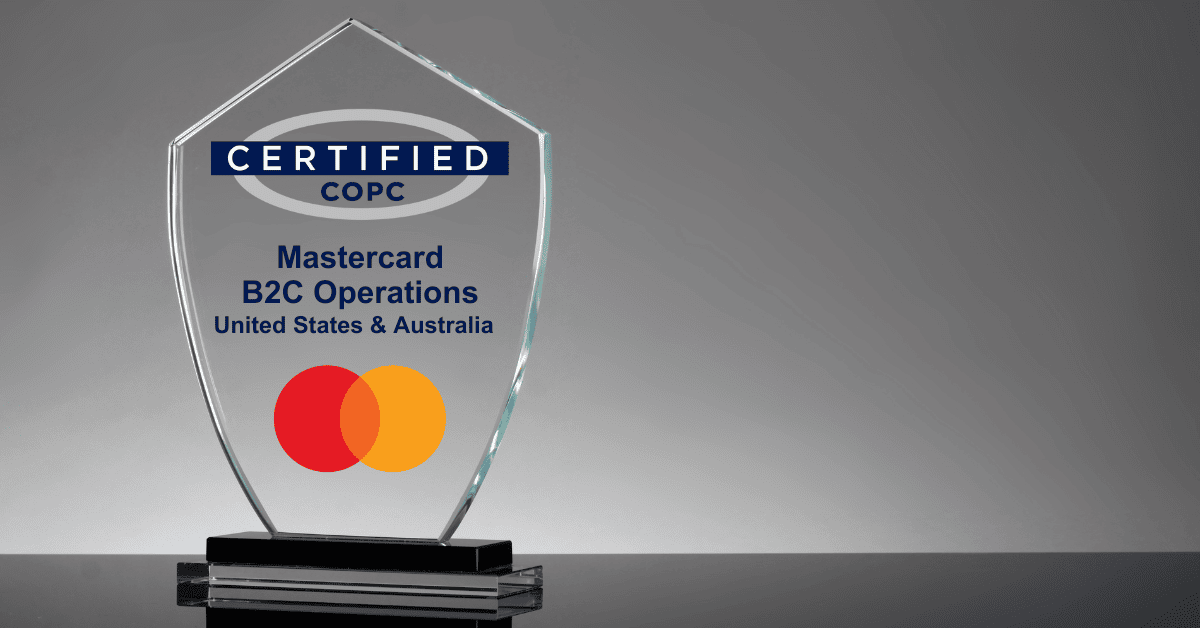
January 9, 2024
Mastercard Leads the Charge in Customer Experience
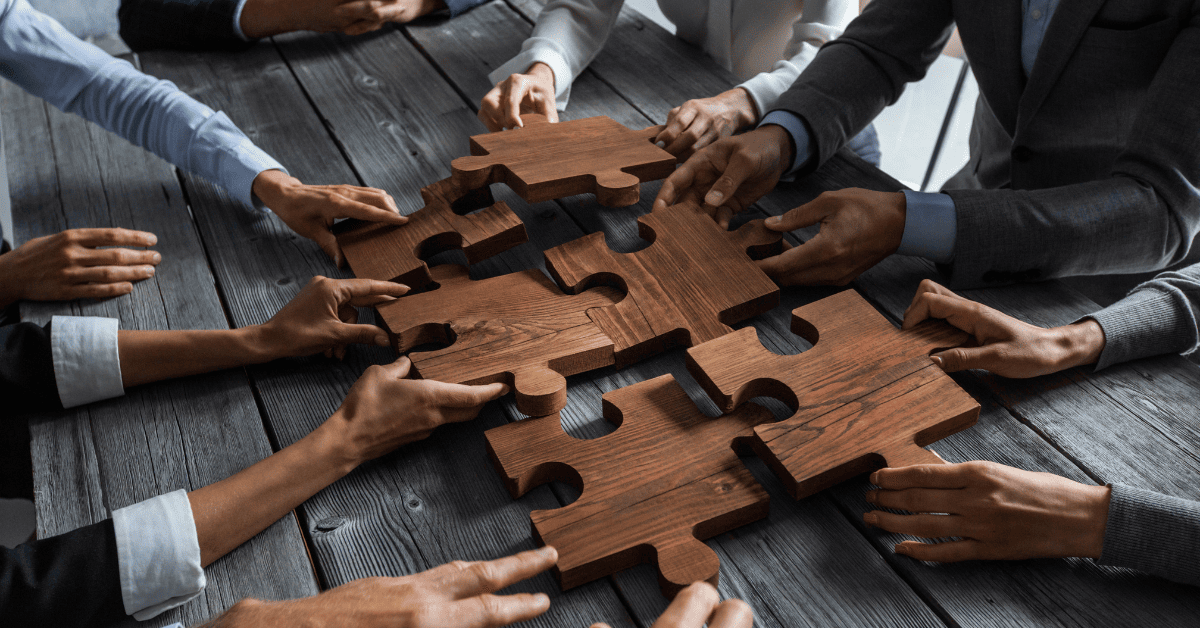
November 29, 2023
6 Ways to Translate Company Values into Employee Engagement
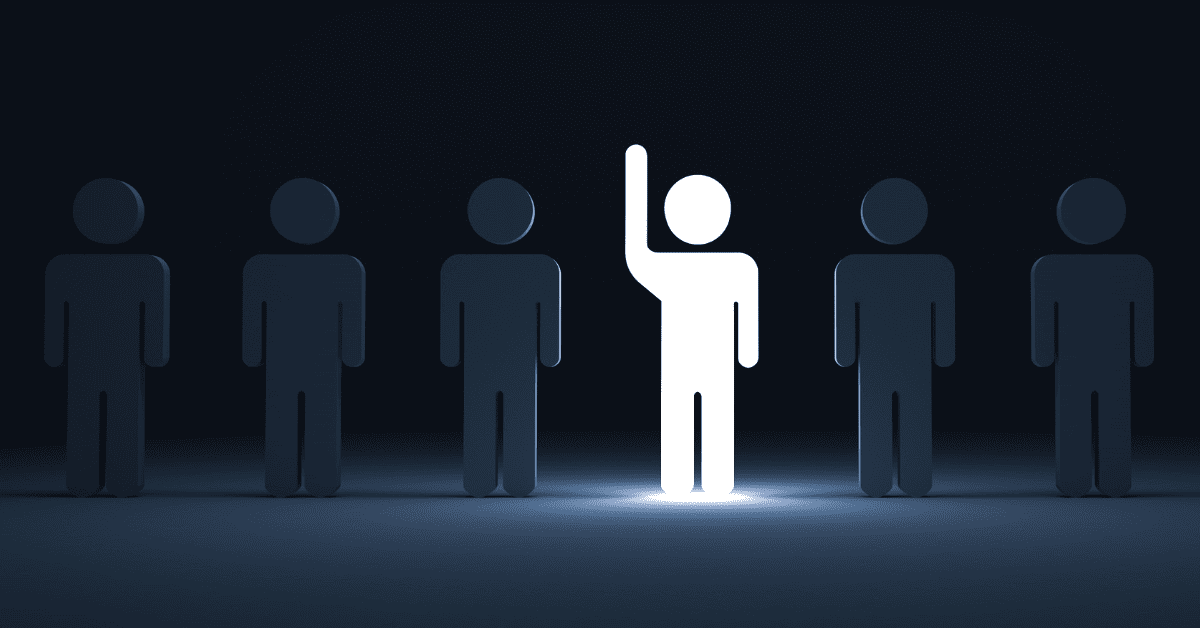
November 15, 2023
Call Center Attrition: Avoid the Dilemma of First-Day Ghosting
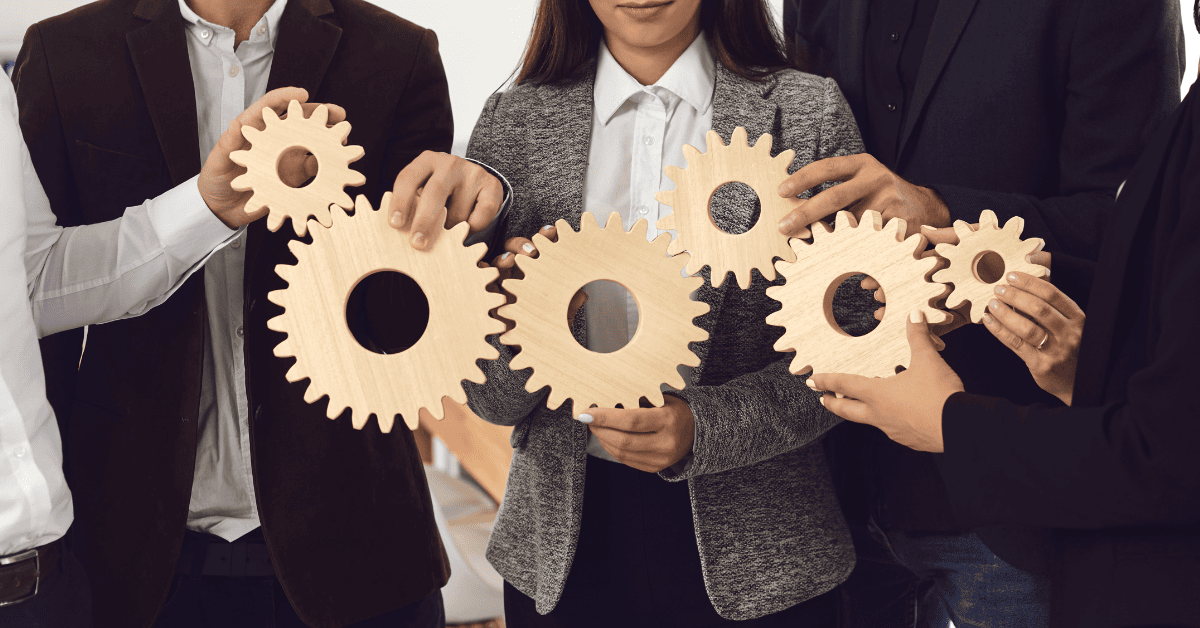
November 14, 2023
The Role of Company Culture in Employee Engagement
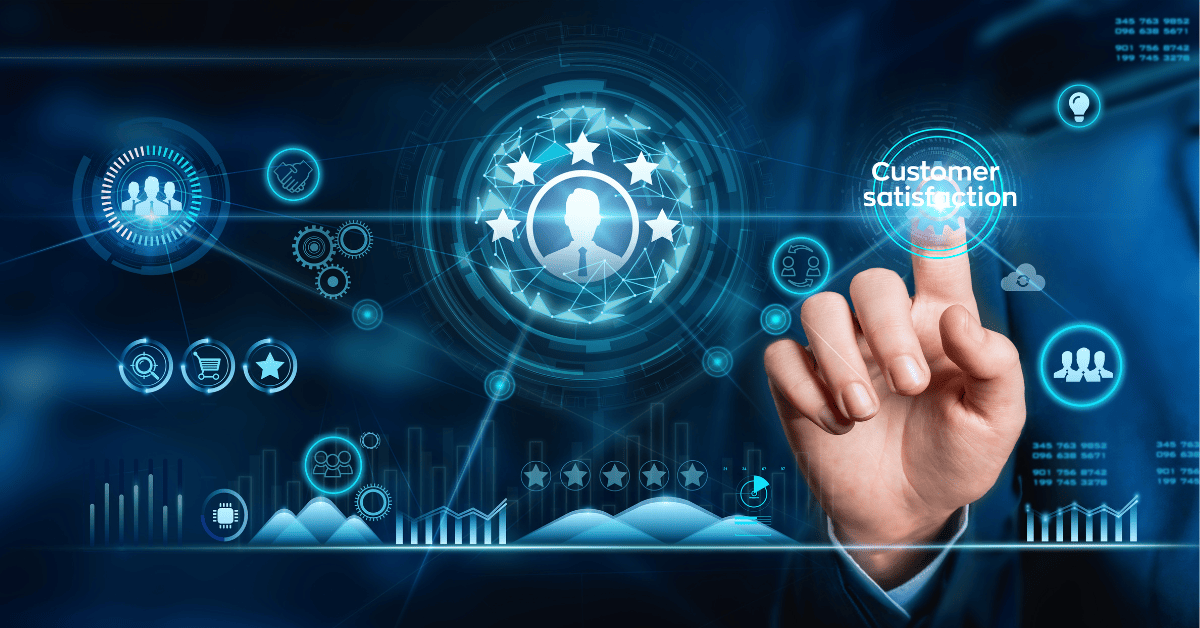
October 24, 2023
The Science Behind Customer Satisfaction (CSAT) Improvement: Finding the Key Drivers
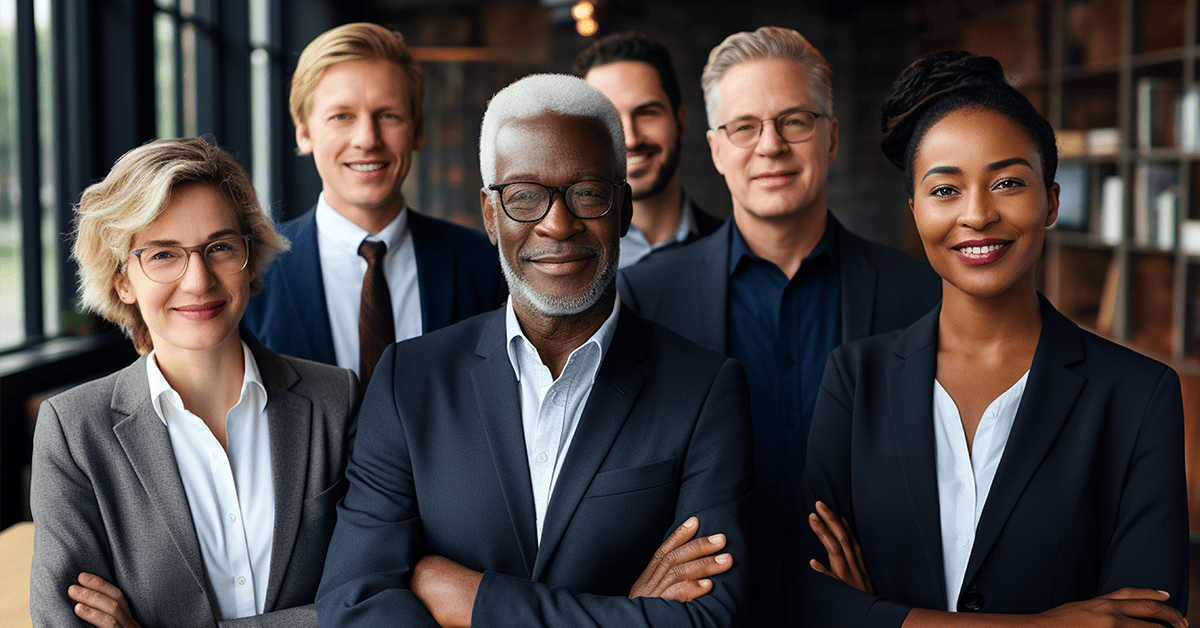
October 16, 2023
Navigating Generational Differences in the Workplace
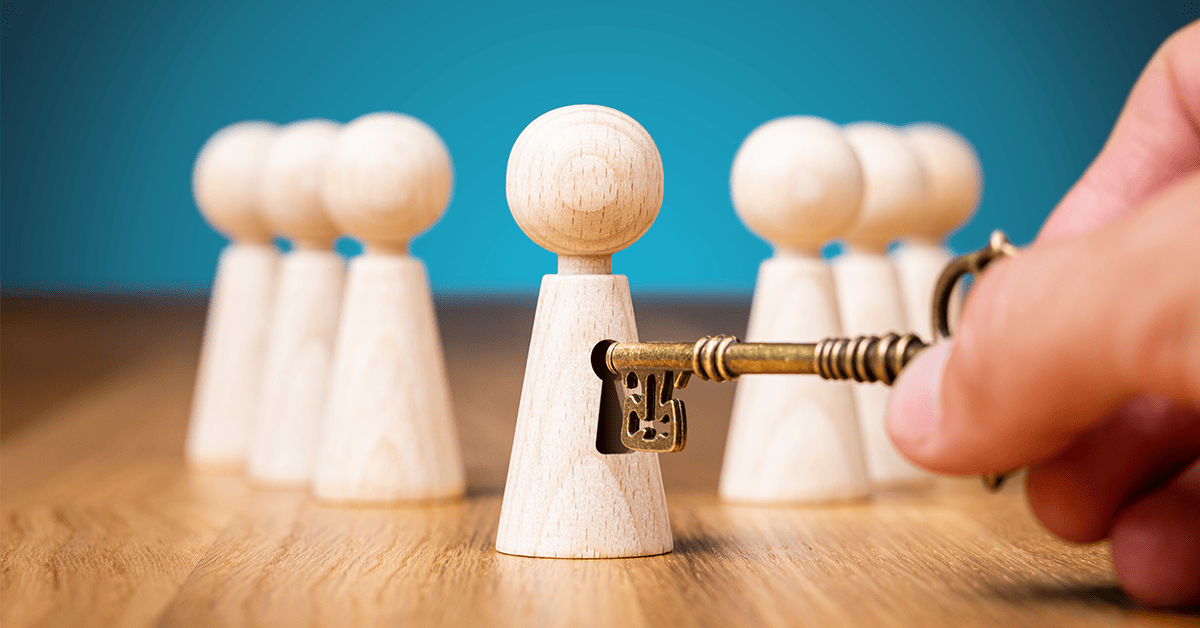
September 21, 2023
Contact Center Coaching to Improve Customer Satisfaction (CSAT)
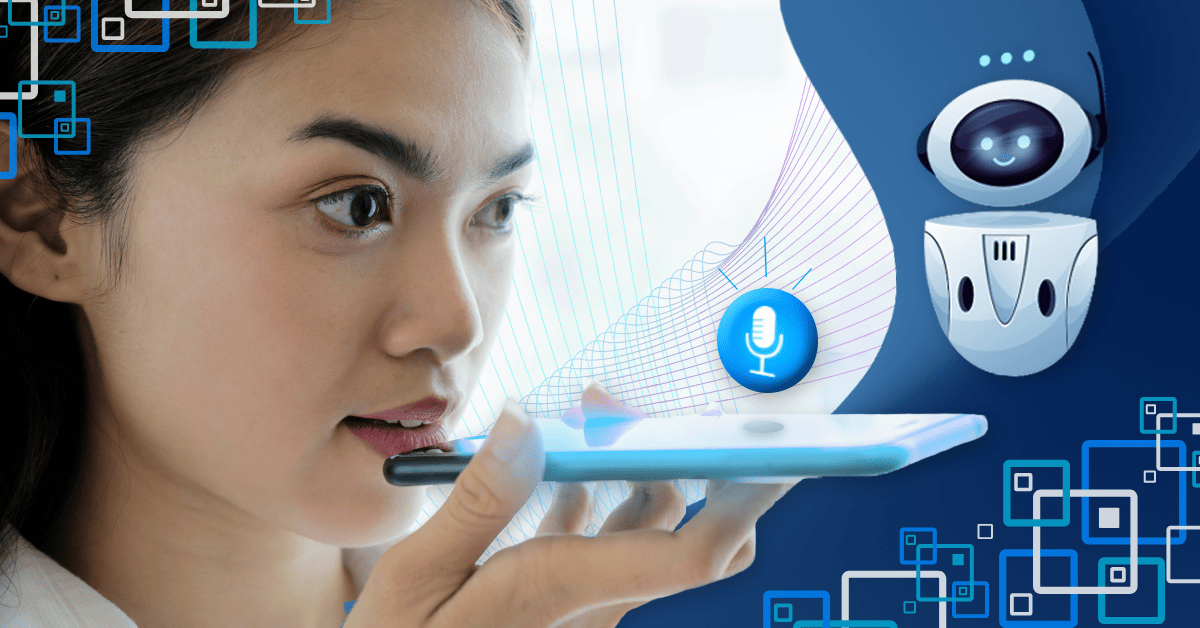
September 11, 2023
A Foundation for Exceptional Digital Self-Service Design
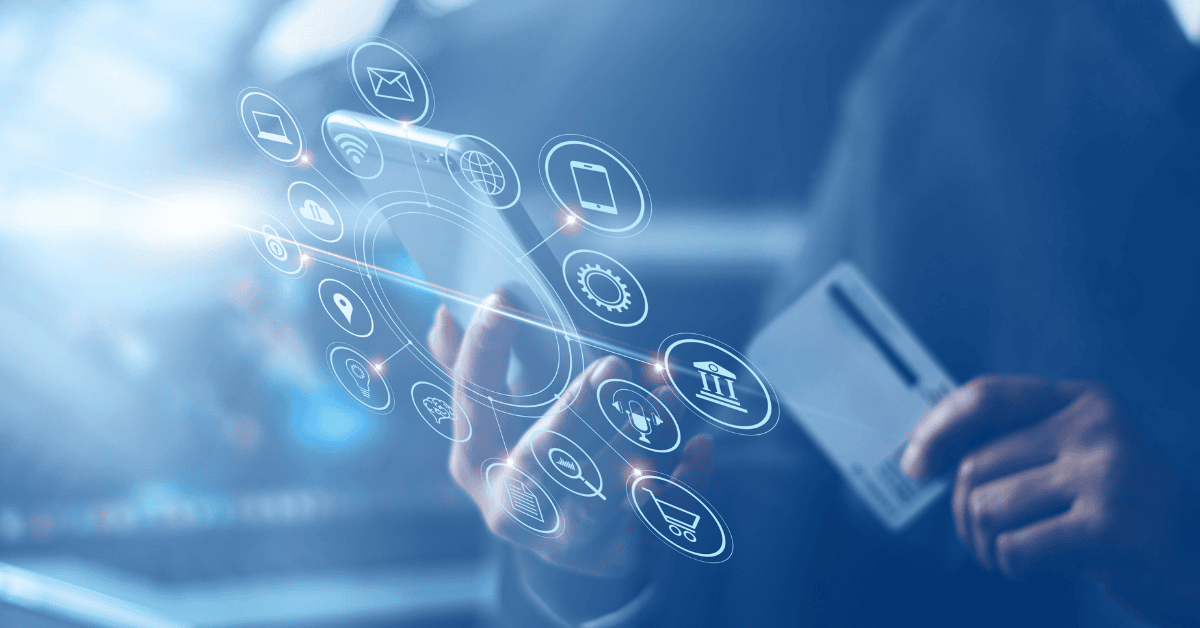
September 11, 2023
Using Channel Strategy to Influence Customer Satisfaction
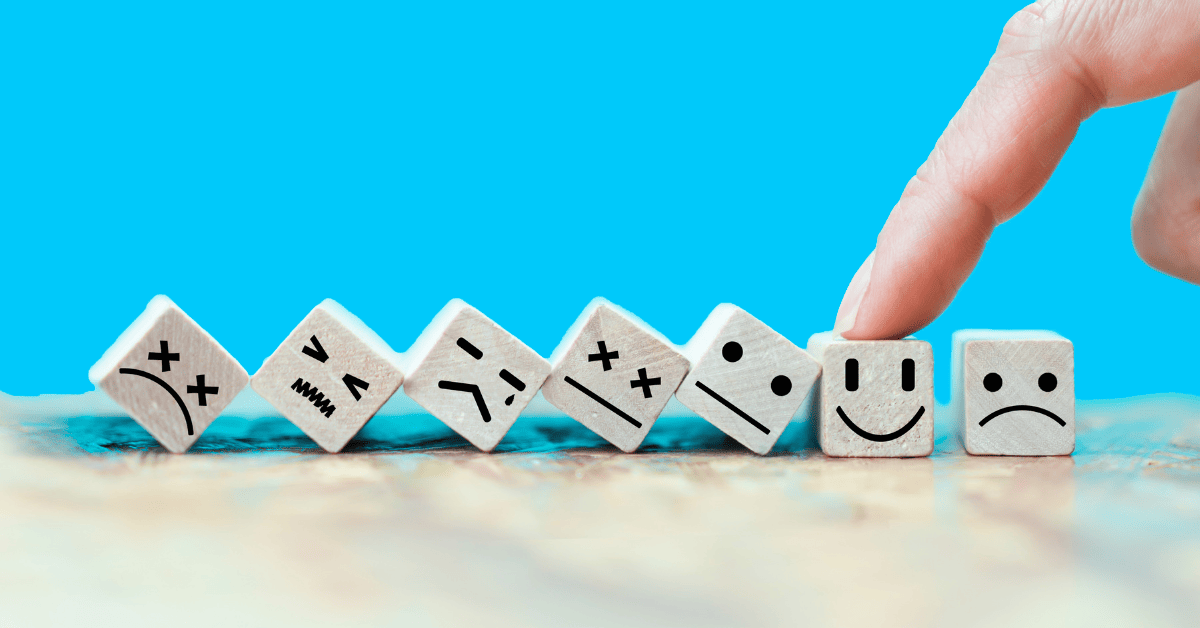
May 19, 2023